Bernoulli, Binomial, Poisson#
Introduction#
Several probability distributions have been studied in depth. Templates like this allow us to model data generated from an experiment quickly. Random variable templates are so useful that several programming languages have optimized how to compute probabilities, expected values, and higher moments.
We will study parametric distributions for random variables. A set of parameters—constants—determine how our random variable assigns probabilities to outcomes.
Discrete Distributions#
The Bernoulli Distribution#
The Bernoulli distribution assigns probabilities to a random variable whose support contains the values 0 and 1. The Bernoulli distribution has a single parameter, often denoted \(\theta\), that controls how probabilities are assigned to these two values, 0 and 1.
To communicate that a random variable \(Z\) has a Bernoulli distribution with parameter \(\theta\), we write:
The parameter \(\theta\) can take any value between 0 and 1 inclusive, or \(\theta \in [0,1]\). The allowable values a set of parameters can take is called the parameter space.
The support of \(Z\) is \(\text{supp}(Z) = \{0,1\}\), and the probability mass function for the random variable \(Z\) is:
We can use the probability mass function to compute the expectation:
And we can use the probability mass function to compute the variance:
Example: Define \(Z \sim \text{Bernoulli(0.45)}\). Then:
Example: A clinical trial enrolls patients and follows them for one year. The clinical team wants to understand the proportion of patients that experience or do not experience an adverse event. We could model whether each patient either experiences or does not experience an adverse event using a Bernoulli distribution. Define \(Z_{i}\) as a Bernoulli-distributed random variable for the \(i^\text{th}\) patient in the study. When \(Z_{i} = 1\), the \(i^{\text{th}} \) patient experienced an adverse event; otherwise, \(Z_{i} = 0\).
The Binomial distribution#
A random variable \(X\) distributed Binomial\((N,\theta)\) has as support \(supp(X) = \{0,1,2,3,4,5,\cdots,N\}\), and the probability mass function is
where \(\binom{N}{x}\) is called a binomial coefficient and is defined as \(\binom{N}{x} = \frac{N!}{x!(N-x)!}\). The binomial coefficient is often read “N choose x” and counts the number of ways one can choose \(x\) items from a set of \(N\) items where the order that the \(x\) items is chosen does not matter. For example, \(\binom{10}{4}\) counts the number of ways to choose 4 items from a set of 10 items where the order we selected each of the four items does not matter.
The expected value and variance of \(X\) are
Given N observations, the binomial distribution assigns probabilities to the number of observations that experience an outcome of interest where we assume that the probability any single observation experiences the event is \(\theta\).
Example Imagine we randomize 200 patients in a clinical trial where 100 are enrolled to receive a novel treatment and 100 are enrolled to receive a control treatment. In the treatment group, 10 patients experience an adverse event from the treatment and in the control group 15 patients experience an adverse event. In previous work we found that the probability any one patient experiences an adverse event in the treatment group is 0.02 and in the control group is 0.04. We can define a random variable \(T \sim \text{Bin}(100,0.02)\) that assigns a probability to the number of patients who experience an adverse event and define a random variable \(C \sim \text{Bin}(100,0.04)\) that assigns probabilities to the number of patients who experience an event in the control group.
Relationship between Bernoulli and Binomial distribution#
Define the random variable \(X_{i} \sim \text{Bernoulli}(\theta)\) Then the random variable \(Y = \sum_{i=1}^{N} \sim \text{Binomial}(N,\theta)\).
Intuitively, we can think of assigning a probability to \(x\) successes and \(N-x\) failures from a Binomial distribution as equivalent to the probability of finding \(x\) 1s and \(N-x\) 0s from \(N\) Bernoulli distributions.
Lets look at how we would show that the sum of two Bernoulli distributions equals a \(\text{Bin}(2,\theta)\) distribution and then generalize.
Let \(Y = X_{1} + X_{2}\). Then the support of \(Y\) is either 0, 1, or 2.
The probability mass function for \(Y\) can be broken up into three cases By considering the sample space \(\mathcal{G} = \text{supp}(X_{1}) \times \text{supp}(X_{2}) = \{ (0,0),(1,0),(0,1),(1,1) \}\).
The sum of two Bernoulli random variables can only equal zero if both random variables take the value zero. In other words, only the element \((0,0) \in \mathcal{G}\)
The sum of two Bernoulli random variables can only equal two if both random variables take the value zero. In other words, only the element \((1,1) \in \mathcal{G}\)
The sum of two Bernoulli random variables can equal one if either the first ranomd variables equals one and the second zero, or vice-versa. In other words, the set of elements \(\{ (1,0), (0,1)\} \subset \in \mathcal{G}\)
The Poisson distribution#
If a random variable \(X\) has a Poisson distribution then the support of \(X\) is all non-negative integers or \(supp(X) = \{0,1,2,3,4,...\}\), and the probability mass function is
where \(x!\) is read “x factorial” and is defined as
For example, \(5! = (5)(4)(3)(2)(1) = 60\). The parameter space for the single parameter \(\lambda\) is all positive real numbers or \(\lambda \in (0,\infty)\).
The expected value and variance are
A random variable that follows a Poisson distribution often corresponds to an experiment where the quantity of interest is a rate. A Poisson random variable assigns probabilities to the number of occurrences of an event in a given time period.
Example The owner of a cafe wants records the number of espressos they produce each day and wants to characterize the probability they produce 0, 1, 2, etc. espressos. For one month the owner records the number of espressos produced per day and find on average that they produce 25 per day. We can model the number of espressos per day as a random variable \(X \sim Pois(25)\).
Relationship between Binomial and Poisson distribution#
Define an interval of time from 0 to \(\tau\) and suppose you wish to count the number of times some event occurs in this time interval. Divide the time interval \([0,\tau]\) into \(\delta t\) equally spaced pieces. Then there are \(N = \frac{\tau}{\delta t}\) pieces from 0 to \(\tau\).
Define a Binomial random variable \(Y \sim \text{Binomial}(N,\lambda \delta t) \) Then we can write down the probability mass function, pull apart each term, and reform the pmf to look similar to the pmf for a poisson distribution.
First we write down the pmf for \(Y\).
Next, lets expand the binomial coefficient
We know that the poisson pmf has the term \((\lambda)^{y}/y!\). Lets re-write our above pmf to look close to this term.
At some point we will ask what happens to this pmf as \(\delta t\) shrinks towards zero. When \(\delta t\) shrinks towards zero we know that the number of pieces our interval is divided into will grow towards infinity. In other words \(\delta t \to 0 \implies N \to \infty\).
Lets try to isolate out terms with \(N\) or with \(\delta t\). First we focus on the \((1-\lambda \delta t)\) term.
Lets look at the term
Because \(N = \frac{\tau}{\delta t}\) we know that \(\delta t = \frac{\tau}{N}\).
We can also work on this term \(( 1 - \lambda \delta t )^{N}\) and see that
Lets plug this in
Now let \(\delta t\) approach zero. This means that the number of pieces \(N\) from 0 to \(\tau\) will increase towards infinity.
Then the term
Not much can be done for the term
As the number of pieces goes towards infinity the size of these pieces \(\delta t\) goes to zero and so
The final term is
and in the limit this approaches an exponential function (see the mathematical aside below if interested in why this limit approaches the exponential function).
This mean then that the probability mass function for \(Y\) converges to the function
In other words, the distribution of the random variable \(Y\) converges to a Poisson distribution with parameter \(\lambda \tau\).
Lets look at an example where we may start with a Binomial distribution but instead decide that the Poisson distribution, while an approximation, is good enough.
Example Suppose we wish to study the number of infections per month for a seasonal infectious agent during the “off-season”. From lab reports we find that there are 1000 individuals who are susceptible to infection. We find further that the probability of infection is a constant \(0.05\) per week and it is likely that \(20\) individuals are currently infected.
We decide to model this as a Binomial distribution with \(N=980\) and probability \(p=0.05\). Then our probability mass function looks like
from scipy import stats
import numpy as np
fig, axs = plt.subplots(1,2, figsize=(8,3))
ax = axs[0]
#--first we will display the pmf for the Binomial corresponding to infections per week
support = np.arange(10,100)
Y = stats.binom( n=980, p = 0.05 )
ax.plot(support, Y.pmf(support))
ax.set_ylabel("Probability")
ax.set_xlabel("Number of infections per week")
#--simulation of three binomial distributions added up.
#--In other words, the total number of infections per month.
infect_per_month = []
for nsim in range(2000):
threeweeks = np.random.binomial(980,0.05,size=3)
threeweeks = sum(threeweeks)
infect_per_month.append(threeweeks)
ax = axs[1]
ax.hist(infect_per_month, 15, density=True, label="Simulated Binomial for three weeks")
#--Then we will display the pmf for the Poisson corresponding to infections per week ( 980 times 0.05 per week times three weeks )
support = np.arange(100,200)
Z = stats.poisson( 980*0.05*(3./1) )
ax.plot(support, Z.pmf(support), label="Poisson approximation")
ax.set_ylabel("Probability")
ax.set_xlabel("Number of infections per month")
ax.set_ylim(0,0.045)
ax.legend(loc="upper center")
fig.set_tight_layout(True)
---------------------------------------------------------------------------
NameError Traceback (most recent call last)
Cell In[1], line 4
1 from scipy import stats
2 import numpy as np
----> 4 fig, axs = plt.subplots(1,2, figsize=(8,3))
6 ax = axs[0]
8 #--first we will display the pmf for the Binomial corresponding to infections per week
NameError: name 'plt' is not defined
Mathematical aside
The goal of our mathematical aside is to show that \( \lim_{N \to \infty} (1+\frac{x}{N})^{N} = e^{x}\). If we can show that then we can see that
One approach is to evaluate the above sequence
for the first couple of values and observe a pattern. For \(N=2\), we find
The above formula is called the Binomial expansion. The Binomial expansion says
We can apply this rule for our problem. Our “\(y\)” is the value \(1\) and our “\(x\)” is the value \(\frac{x}{N}\).
Lets see if we can make sense of the first few terms and find a pattern.
It looks like we will end up with terms like \(\frac{x^{n}}{n!}\) and then a product of terms like \( (1-1/N)(1-2/N)(1-3/N)\) etc. Because each of these terms goes to one as \(N\) goes to infinity their product will go to one too. Then we’re left with
This is the series expansion for \(e^{x}\). In other words
Relationship between Bernoulli and Poisson distribution#
Again, suppose we ask to compute the probability that a specific number of event occurs in an interval \([0,\tau]\). We may decide to model the number of events that occur in this interval using a Poisson random variable. For example, we can define a random variable \(X \sim \text{Pois}( \lambda \tau )\). Where \(\lambda\) is a rate (number of occurrences divided by time) and \(\tau\) is a time interval.
The pmf for \(X\) is
and the probability of no occurrences is
and the probability of a single occurrence in this interval is
Again, partition this interval from 0 to \(\tau\) into small pieces of length \(\delta t\). Then the number of occurrences in the interval of length \(\delta t\) is
where the random variable \(Y_{1}\) is the random variable corresponding to the first interval \([0,\delta t]\). But if we assume that \(\delta t\) is very small, we can approximate the probability assigned to zero occurrences and one occurrence. We will see that this approximation lends itself to defining a new random variable that is distributed Bernoulli.
We know that the exponential function has a series representation
However, if \(\delta t\) is extremely small then \((\delta t)^{2}\) is so small it can be excluded. That is
Lets plug-in this approximation for our random variable \(Y_{1}\).
The probability of any occurrences beyond one is so small that is negligible as well.
We can define a random variable \(Z \sim \text{Bernoulli}( \lambda \delta t )\) that represents this approximation. That is, if we cut the interval \([0,\tau]\) into \(N\) pieces of length \(\delta t\) then we can define \(N\) Bernoulli random variables with parameter \(\lambda \delta t\) and
interval = (0,10)
interval = interval[1] - interval[0]
deltat = 1./20
rate = 5
bern_approx = []
for nsim in range(2000):
s = 0 #<--Sum of bernoullis
for _ in range( int(interval/deltat) ):
s = s+ np.random.binomial(1, deltat*(rate) )
bern_approx.append(s)
plt.hist(bern_approx,15,density=True)
support = np.arange(30,70)
Y = stats.poisson( rate*interval)
plt.plot( support, Y.pmf(support) )
[<matplotlib.lines.Line2D at 0x115b411d0>]
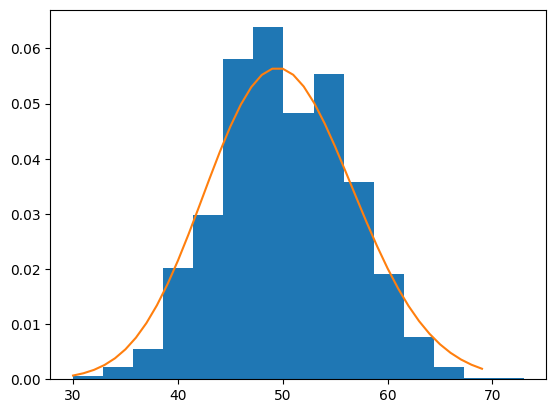